Artificial intelligence may seem as if it has come from nowhere to dominate conversations about the future of work in just a couple of years, but most financial institutions have been using it in some way or another for a long time.
So far, some of the most common and most fruitful use cases of machine learning and traditional AI, as some describe it, in the financial sector have been in fraud detection, anti-money laundering, surveillance and transaction monitoring, profiling of marketing data and propensity modelling.
What has changed more recently is the introduction of foundation models, language models and generative AI.
Artificial intelligence, broadly, is the use of computational models to do complex work that could previously only be done by people. In traditional AI, the tasks the machine performs are executed according to pre-set rules. The machine can reason logically and can conduct complex if repetitive and well-defined tasks with structured data, but it requires human intervention to define the rules under which it operates.
Generative AI can create its own rules based on the large, unstructured datasets it learns from and can create its own ideas and solutions. It is more flexible and better able to adapt without intervention.
“If you had asked a year ago to any financial institution if they are using internal co-pilots, almost none would have said yes, but there has been a massive ramp up in the last six months,” says Jacque Theys, department head of advanced analytics and business intelligence at Euroclear, the Brussels-based clearing house.
“We’re very active on the topic,” says Andrei Serjantov, head of digital markets at BNP Paribas. “It is absolutely a given that gen AI is going to play a big role in global markets. We see a lot of uses of this technology.”
Firms in the capital markets are prime contenders for gen AI experimentation. “Investment banks are environments where you have to be fast to use these tools if they provide a competitive advantage; you need to be aware of what it brings and leverage it,” says Theys. “Virtually anything can be automated.”
“A bank today cannot operate without AI,” says Jose Moreno, partner and lead of the centre of excellence in artificial intelligence, advanced analytics and cognitive solutions at EY in Madrid. “Almost every single model that runs in banks runs on traditional AI. Indeed, financial services is probably one of the sectors where AI is most used.”
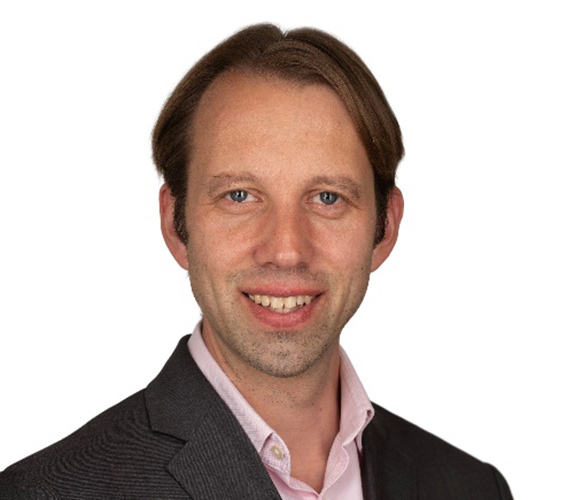
However, while banks have deployed machine learning readily in back and middle office tasks — largely administrative work — they are cagier when it comes to admitting to the use of AI in the risk taking or client facing work done in the front office. A number of investment banks declined GlobalCapital’s request for a comment about their use of AI in the capital markets, for example.
“Anecdotal evidence we have seen so far [suggests] that the application of AI [in the capital markets] is, by and large, concentrated on increasing the degree of automation in back and middle office tasks,” noted Johannes Kramer, senior financial economist at the International Monetary Fund, speaking at an Association for Financial Markets in Europe (Afme) conference in November. “Our impression was that firms oftentimes downplayed AI usage in front office trading, potentially due to regulatory or reputational concerns.”
But patent filing and job advertisements suggest growing interest in using AI for front office roles, he said.
Efficiency plays
Over the past year, most AI deployment in financial services has been to improve a company’s internal efficiency. At a roundtable in January, Serjantov said that across the BNP Paribas group, there were more than 700 AI use cases and more than 25 gen AI use cases in experimentation. These numbers are increasing all the time.
BNP Paribas backed French start-up Mistral AI, with the bank taking part in two investment rounds in the company over the past year. In July, the bank announced a partnership with the firm to use all of its models across its whole business.
Serjantov says gen AI is applicable in code generation, translation and information extraction.
“It’s everything where operational efficiencies can be reaped and always taking into account what the risk is if you get it wrong,” says Frank De Jonghe, Europe, Middle East, India and Africa financial services quantitative and analytics leader at EY. “I think that’s the hardest trade-off in a lot of these things.”
“If a prospectus is 40 to 50 pages, and we get thousands of prospectuses a day, you get a decent amount of homework,” says Euroclear’s Theys. Using generative AI for this use case is common practice across the market, he adds.
Information extraction is a focal point for many financial firms. A lot of information in the financial services is kept in emails, in PDF documents or is taken from earnings calls, for which different companies have different structures and templates.
“There’s a tremendous amount of information locked away in unconventional sources,” says Emily Prince, group head of analytics at the London Stock Exchange Group (LSEG). “Now we have a cost-efficient way of extracting and identifying patterns in that information.
“If you’ve ever tried asking 1,000 people to do the same task, you’ll know the results can vary widely due to differences in experience, productivity and education. Working alongside… domain experts, AI offers opportunities for greater consistency and reduces the need for extensive refinement.”
The IMF suggests in its global financial stability report, published in October, that there is evidence AI could make markets react faster to news. IMF staff tested the theory on US Federal Reserve meeting minutes, a usually complex and lengthy document. The data suggests, according to the IMF report, that after the introduction of large language models (LLMs), “the initial market reaction following the release of the minutes (up to 45 seconds) tends to reflect its eventual impact more accurately than in the period before the introduction of these technologies”.
The next step is for AI to start automating front office work, including deal execution. “I think for 2025, and certainly the next two or three years, the most significant advances in technology will come in automation,” said Tim Whipman, head of business development at TransFICC, a trading technology company, speaking at the same Afme conference as the IMF’s Kramer.
The use of the word automation here is notable as it means something new when it comes to gen AI. It used to refer to straight-through processes and back office operations, but “that is not what it means at all anymore”, said Whipman.
“We’re talking about having been through a decade or two of electrification of different fixed income markets. We’ve now moved to a phase where the sell-side and the buy-side need to be able to automate not just their post-trade processes, not just the integration of market data, but the execution process as well. That can be extremely complex.”
Unprecedented uptake
The early feedback suggests that deployment of gen AI tools has been a success, even if it mainly concerns handling the daily grind. “The feedback from the employees has been unprecedented,” says Serjantov at BNP Paribas. “The take-up from employees has been tremendous. People see this as something that helps them with their most boring, most mundane, most repetitive tasks.
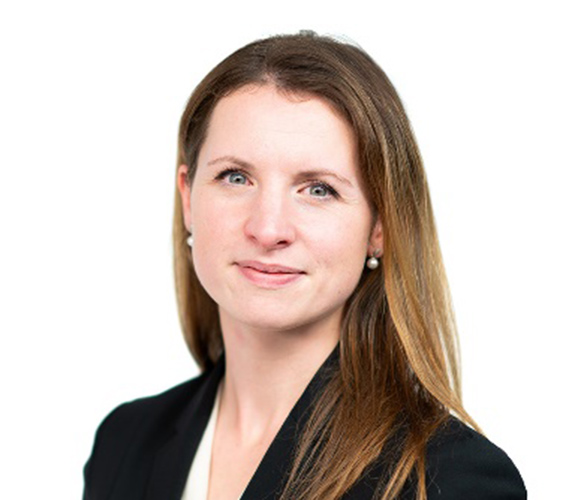
“We’ve run surveys and looked at external publications from Microsoft to Accenture and have found satisfaction rates in the 90%.”
Although use of the bank’s internal AI tools varies depending on the task, active regular users of the French bank’s LLMs use it multiple times a week, says Serjantov.
“Gen AI is really becoming part of the day to day of [EY],” echoes Theys. “Employees use it on a regular basis every day,” referring to Microsoft’s Office 365 embedded Copilot, which Euroclear rolled out for internal use this year.
An AI expert at a trading venue says employees are “increasingly” making use of internal AI tools like co-pilots and Q&A systems.
Euroclear has run training sessions so its employees can use the technology to its fullest extent within the company’s framework, making sure no public, external generative AI tools are used. But of course, some users are more enthusiastic about it than others. “Some people won’t use a copilot before they retire and some people use it from day one,” says Theys.
Big to small
The development of gen AI started with a race towards very large language models such as ChatGPT, launched in November 2022, and Gemini, launched in December 2023.
But although the classic advantage of gen AI is that it can learn from large, unstructured data sets, some are training it on specific data to solve specific problems.
The industry has sprouted a proliferation of small language models, which are computationally lighter. There is increasing diversification and specialisation.
“We have been deploying many use cases for operations, namely, automatic processing of documents, email analyses and internal chatbots,” says Theys, adding that a generative AI copilot tool specifically trained on Euroclear’s own internal legal data has been deployed for the legal teams, for example.
Since the release of the internal legal team’s gen AI tool, which was launched at the start of this year, “we have been getting a lot of interest from other departments,” says Theys.
His department is now in the process of collating the internal demand and preparing a roadmap for the coming months, prioritising which teams would most benefit from a copilot. Among those is the company’s risk team. “Our risk colleagues can already use this to build reports for management,” he says. “Based on the data they have, they can pre-draft some reports using generative AI.”
Interest in AI tools is also growing at BNP Paribas. Whereas the bank’s AI team previously had to pitch the technology to colleagues, that has flipped. “Before, [the AI team] would have to ask business teams whether they had any problems solvable by AI, but this is completely over,” says Serjantov. “Now, it’s the business teams that are knocking on the doors of the data science teams.”
Clients next
Some financial services firms have gone a step further by offering gen AI tools to clients.
The LSEG has “collaborated extensively with… customers to develop AI products, incorporating their feedback and establishing a robust framework, supporting safe and scalable innovation,” says Prince.
The LSEG has launched external tools for code generation to clients over the past few months. The LSEG’s Lipper AI Insights tool, for example, allows clients to query data on investment funds.
“A client might ask: ‘How have emerging market funds performed over the past year?’ or ‘What’s the historical performance of this specific fund?’” says Prince.
While the first rollout has been around funds, the use will be extended in the future.
UBS Group has developed an AI tool to help it offer clients M&A ideas, producing analysis of a database of over 300,000 companies in less than half a minute. It went live in September.
The bank built its M&A copilot to generate target ideas for buyers as well as to help sellers find possible acquirers, Brice Bolinger, UBS head of M&A Switzerland, said at a conference in Zurich in September.
UBS declined GlobalCapital’s request for further comment on its M&A copilot and other uses of AI.
Not all, but most of BNP Paribas’s uses of AI are internal. The bank declined to comment on external use cases.
Train rather than hire
The first stage for any financial market player is to staff up and develop technical knowhow about AI. “We’ve been hiring a lot of data scientists to start leveraging more use cases,” says Theys, adding that a hiring spree of data experts is underway at many financial institutions this year.
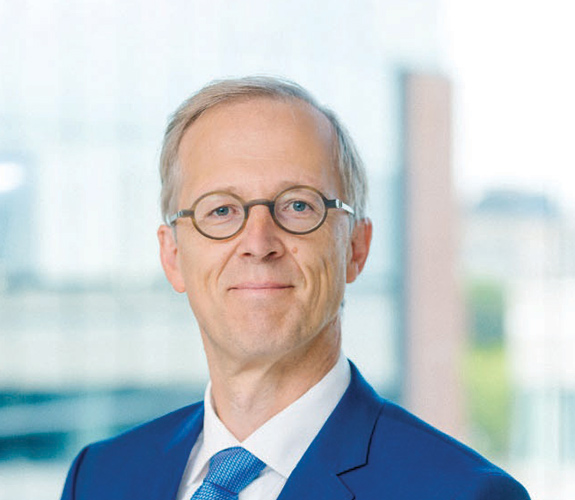
It is crucial to implement robust governance and control measures, irrespective of the different legislations that may apply
But the industry is now moving to a second stage, focused on providing AI training to a broader population within businesses. “The ideas and business cases for AI will come from those people — those who know the business and who are facing the day-to-day problems,” says Theys.
Indeed, market participants emphasise the fact that, while machine learning engineers and AI experts are important, the real emphasis is on training staff with financial expertise in the use of AI.
“There is a sense that you need machine learning engineers to approach the problem,” says one AI expert, “but to be honest in my experience you need your domain experts — people who really understand a bond prospectus or really understand a financial instrument.”
“There’s sometimes a perception that machine learning engineers alone can solve these challenges,” agrees Prince. “To achieve accuracy, you also need domain experts working alongside engineers and data scientists.”
“There is no substitute for getting people experienced with these models,” adds Serjantov.
AI algos
AI is yet to make its mark on trading, however, although market participants believe its use will grow quickly.
At the Afme conference in November, an audience poll showed that up to 25% of electronic trading flows are steered by AI-driven algorithms. “I wouldn’t be surprised if it’s actually more towards the zero than the 25%,” said Whipman.
When asked what degree of autonomy the audience expect AI-based algorithmic trading to reach in the next three to five years, 84% opted for semi-automated execution with human oversight for critical decisions and 16% said there would be a minor role for AI with human oversight.
None thought there would be no role for AI or that algorithms would be fully automated with little to no human oversight.
The growth of AI in trading is likely to resemble evolution rather than revolution. “The way they’d see [AI in trading] evolving was very much that this isn’t something new for [them], this is just an extension of today’s algos,” said Whipman. “Today’s machine learning tools [are] just going to be something that will make the market even faster, more liquid.”
But for some financial institutions AI isn’t just an enhancement, it’s the foundation of their entire business model. This is the case for many quantitative research firms.
One says it has eliminated the need for human traders entirely, claiming every trade it makes is executed by AI systems. The firm operates with a lean team of machine learning and AI experts, whose sole responsibility it is to train LLMs and algorithms to make decisions.
Indeed, in the world of quant finance the need for people with financial expertise is no longer of central interest to recruiters. Recruitment marketing materials from leading quant firms say that traditional finance backgrounds are unnecessary. Instead, they want people at the cutting edge of machine learning.
A second quant firm also operates on an almost entirely AI-driven business model and a third has this year started developing a subsidiary of its business to be completely run by AI, with the ultimate ambition of transitioning its entire trading operation to AI, said a source familiar with the firm.
For these firms, AI is not just a tool to solve particular problems or to automate a few processes, it’s the core of their strategy.
For banks, handing over the keys to the trading floor to a machine is some time away. “I wouldn’t go there yet”, says Serjantov, “because something like that would take a tremendous amount of investment and is questionable whether you’d actually want to do it.
“On a risk-reward basis, there’s so much stuff out there to get done before you start pushing the envelope to something like that. We can get a huge amount of value using some of these models on some much simpler use cases.”
Whipman, perhaps unsurprisingly, sees the need for urgency rather differently. “We’re seeing higher trading volume and potentially smaller ticket sizes in some fixed income asset classes, and… for banks to be able to respond to this increase in volume and more complex workflows… they have to automate,” he told the Afme conference.
“If you want to trade a government bond or a credit bond or any fixed income instrument on a venue, the coding to APIs is complex, workflows are numerous and there are new ones coming all the time. To be able to automate that process and normalise those API-supported venues is extremely important.”
An API, or application programme interface, is a connection between two software systems that allows them to communicate with each other.
While a survey of 26 banks, including global and regional firms, identified automation as their number one technology priority, Whipman warned that a bank must ensure its trading infrastructure can support AI implantation. “Putting AI on top of poor infrastructure, it’s like putting a Ferrari engine in a Fiat Panda,” he said.
Indeed, some of the quant firms that trade exclusively using AI-driven models invest hundreds of millions of dollars a year in computers. They are building clusters comparable in size to the ones used by tech giants like Meta and Google, says a source familiar with the industry.
Regulating piece by piece
With great computational power comes great responsibility — or at least a few rolls of red tape. Regulating AI is in its infancy, but the EU has gone further than the US or UK by establishing an Artificial Intelligence Act, which was passed into law by the European Council in May. The EU has decided to regulate AI with a single law covering its use in all sectors.
But despite the comprehensive nature of the rules, some operations are not covered. “The EU AI Act primarily focuses on protecting natural persons,” says EY’s De Jonghe. “For example, if an individual is involved in algorithmic or quantitative trading using complex algorithms, which can pose significant risks for an investment bank, the EU AI Act does not specifically address this area. Therefore, it is crucial to implement robust governance and control measures, irrespective of the different legislations that may apply.”
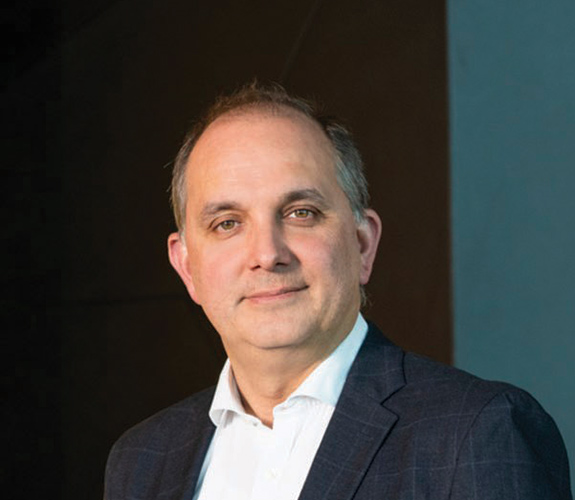
The EU AI Act officially took effect on August 1, 2024, but its provisions will be implemented gradually. None of the requirements apply as of November 2024, with the first prohibitions on certain AI systems starting on February 2, 2025, and the majority will apply from August 2, 2026.
The European Commission is also developing guidelines to define and detail how the Act should be implemented, with the aim of developing standards and codes of practice.
Elsewhere, the National Institute of Standards and Technology, part of the US Department of Commerce, and the Institute of Electrical and Electronics Engineers Standards Association, an international body, are working on AI frameworks, which the EC intends to make use of.
Without clear AI regulation for the financial services industry, regulators rely on AI-using companies responding to infrequent surveys.
“That, of course, heavily relies on voluntary contributions and self-reporting, [which] somehow we felt was lacking regulatory enforcement,” the IMF’s Kramer told the Afme conference. “That inherently limits [regulators’] ability to capture particularly rapid market developments… and identify AI risks in near time.”
Since the 2008 global financial crisis, there has been “significant regulatory fatigue”, said Kramer, adding that, when surveyed, European market participants pushed back against new reporting demands, particularly for emerging technologies like AI.
“There seems to be not a fear but a concern around what regulation is to come, and a lot of these banks feel reluctant to implement these AI strategies at this point,” Whipman told the Afme conference.
Indeed, market participants question how the EU AI Act will interact with heavily regulated bodies such as banks. Banking regulators have raised concerns about a higher regulatory power looking at the models that banks use to do capital calculations and safety and soundness reviews.
It is a tall order. The Commission was understood earlier in the year to have only six to eight people working on the topic and around 80 to 90 tasks to get through.
However, this presents financial firms with an opportunity to take the initiative and show the EC what best practice looks like.
Who’s in charge?
Market participants flag concerns about potential conflicts of supervision. There is not yet “any gap analysis [comparing European frameworks] with UK and US frameworks”, a spokesperson at the European Securities and Markets Authority (Esma) tells GlobalCapital, but there are concerns about how industry-specific regulation should interact with the EU Act.
The EU’s tack has been to take a principles-based approach in the belief that this is the best way to future-proof technology that is moving very quickly.
“The [UK] government has come out and said that they are going to put in legislation as well,” said Alexander Tollast, counsel at Clifford Chance, speaking at the Afme conference.
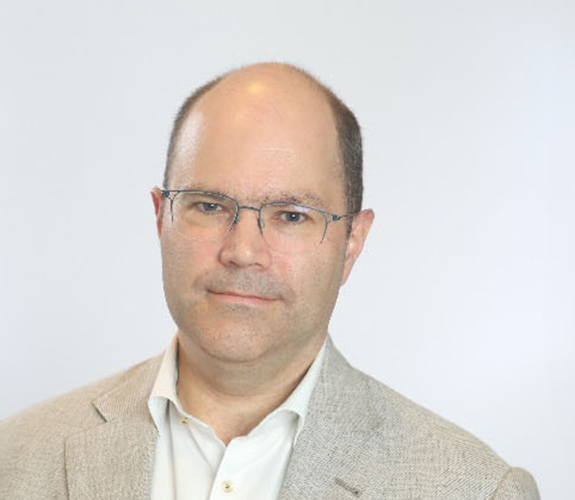
We have an AI expertise team, which governs the what and the how — all AI use cases that want to go live are screened and reviewed there
“Undoubtedly, the British will try to promote something which attracts a little bit more business, so there may be some arbitrage between the EU AI Act and the UK equivalent.”
EU financial regulators have begun providing guidance to financial institutions on their use of AI this year. In May, Esma issued a statement providing initial guidance to financial firms using AI when they provide investment services to retail clients.
“When using AI, Esma expects firms to comply with relevant MiFID II [Markets in Financial Instruments Directive II] requirements, particularly when it comes to organisational aspects, conduct of business and their regulatory obligation to act in the best interest of the client,” says the regulator’s spokesperson. “In 2025, Esma will focus on financial innovation, particularly in the area of AI with the aim of ensuring investor protection and market integrity.”
But what the EU lacks is coordinated regulation. Data protection regulators; the EU’s AI Office, which is its centre of expertise on the topic; the EU AI Act; the enforcement units of the EU’s Digital Services Act and more have been accused of working in siloes. In fact, there has been criticism that there are so many financial services and AI regulators in Europe, that it is unclear who has the final word.
Self-regulating
“Every time we deploy an AI use case or an AI model, we are making sure that it’s classified under the [EU] AI Act,” says Theys. “We want to comply with new regulation that comes out by design. It’s not just a nice to have for us.”
Another regulatory expert says: “If you’re sitting at an organisation, my best advice has always been in the past year to say that you’re responsible for the outcome, irrespective of whatever guarantees the EU AI act might give you. Just assume that you’re responsible for the outcome.”
A lot of the things that are important to financial institutions using AI, like the use of multiple models and understanding model explainability, are already “extremely well served” within existing regulation, says an AI expert. “There are many types of regulations already in place, plus we’ve got extensive model risk management that has been in place for decades.”
“Because we’re in a regulated environment and we’re so used to risk management — whether it’s market risk or operational risk or legal risk, etc. — we’re actually in quite a good position already to make those use cases safe,” says a second expert.
Indeed, the years of post-crisis regulation may have created two tiers in terms of compliance. Banks have by now a more ingrained risk management culture. That is not necessarily true of non-bank financial institutions although Euroclear says its procedures are robust.
“In terms of governance, we have clear cataloguing of all the models we put in production,” says Theys. “We have an AI expertise team, which governs the what and the how. All AI use cases that want to go live are screened and reviewed there.”
In the screening process users must answer whether the AI is something that has to run 24/7 or just during business hours, how critical the model is to the operation, who owns the models, who benefits from the technology and who is responsible for making sure it is maintained and monitored.
“We are prudent in mastering the side effects of a potential AI hallucination or mistakes,” he adds. “As a financial market infrastructure, we cannot afford 1% of error. I don’t think anybody can claim that a model is bullet proof. We make sure to keep a human in the loop in mission-critical cases.”